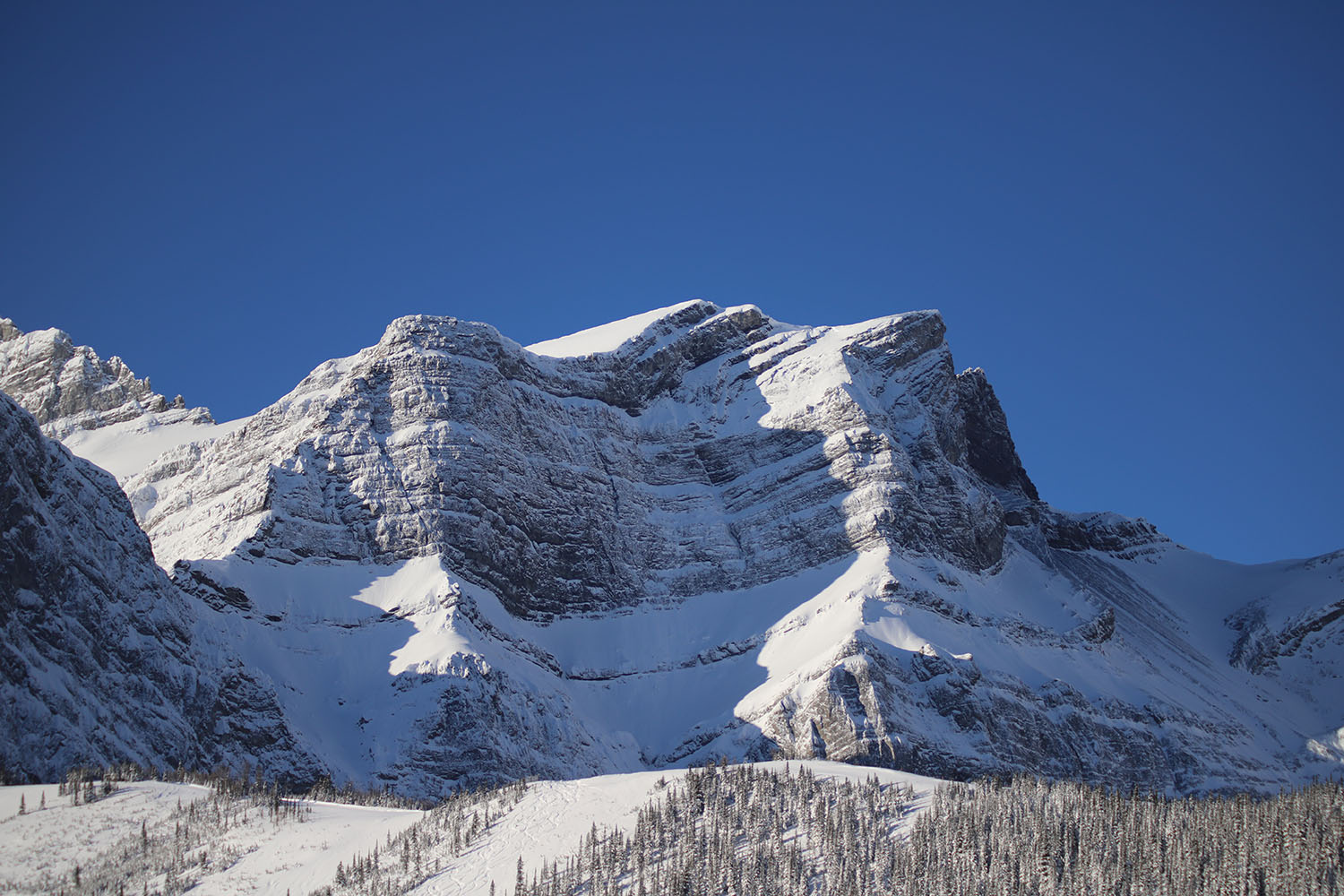
USask model for predicting mountain snowpack provides clearer picture of spring runoff, impacts of climate change
Scientists have developed a way to predict the depth and movement of snowpacks in the Canadian Rocky Mountains which will provide valuable information on spring runoff, risk of flooding, avalanche danger, and the impact of climate change.
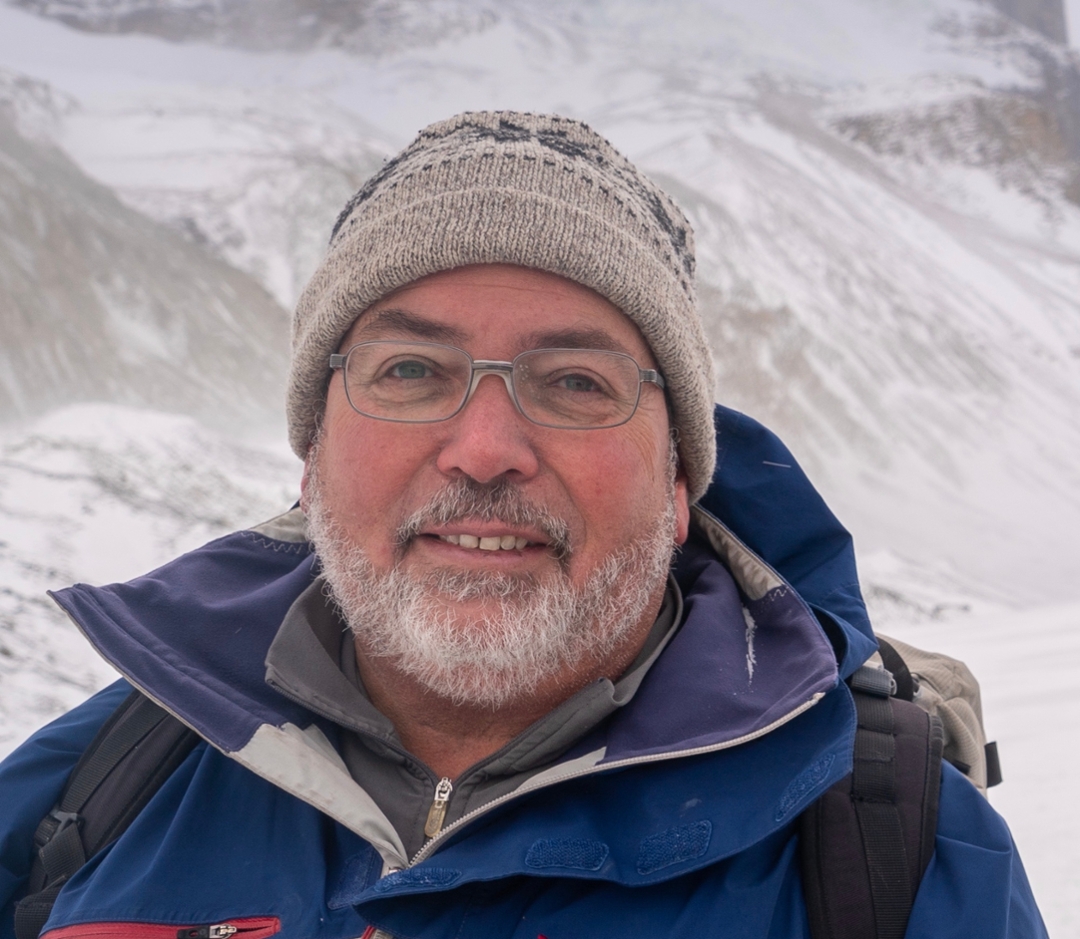
After decades of research, a new model was developed by University of Saskatchewan (USask) researchers that for the first time successfully predicted mountain snowpack with a high degree of accuracy and detail—information of critical importance for water management, agriculture, mining, recreation, and flood forecasting worldwide. Snow cover in the mountains is the principal driver of spring runoff.
Read more on this publication.
“Snow in the Canadian Rockies accounts for 60 per cent of the flow of the South Saskatchewan River and three-quarters of the province relies on it for drinking water, for irrigation, for potash mining and other industries,” said John Pomeroy, Canada Research Chair in Water Resources and Climate Change and professor in the USask Department of Geography and Planning. “The rivers in Saskatchewan really are the lifeblood of the province.”
According to Pomeroy, more than half of humanity relies on runoff from mountain snow for drinking, power generation, and irrigation.
“We can finally predict the development of mountain snowpack,” said Pomeroy. “This is a big achievement, which allow water management agencies to better assess our mountain snow water resources.”
For example, the amount of snowpack in the Rockies each winter determines hydroelectric capacity at the Gardiner Dam, and the amount of water available in Lake Diefenbaker for irrigation.
Big data
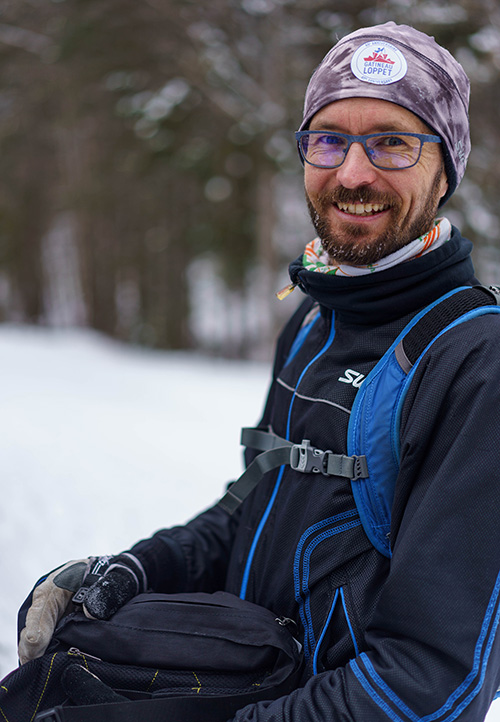
Run on supercomputers, the Canadian Hydrological Model (CHM) crunches detailed data on snow distribution by wind and avalanches, shading by mountains, wind flow over ridges, and vegetation, along with weather forecasts, to generate an estimate of where and how much snow has accumulated in a given area.
The USask team used their model to predict the amount of snowpack in a 1,000-square kilometre area of the southern Kananaskis Valley, in the Canadian Rockies. Their results, published in mid-February in the journal The Cryosphere, were a close match with snow depth data collected by a team of scientists at the University of British Columbia using LiDAR (light detection and ranging) laser measurements taken by airplane. High-resolution snow cover data captured by satellite and processed by a lab at University of Toulouse (France) confirmed the results.
Generating estimates of snow accumulation is currently a costly, labour-intensive exercise, relying on teams of snow surveyors going into the backcountry on skis or by helicopter, then manually measuring snow depth and density in remote locations—techniques that have been used for over a century. Because of cost and time involved, they can only take measurements at a few locations.
The USask team has created a demonstration website called Snowcast which uses their model to generate nearly real-time estimates of snowpack for a section of the Bow Valley starting just west of Calgary and running up to Lake Louise and Field.
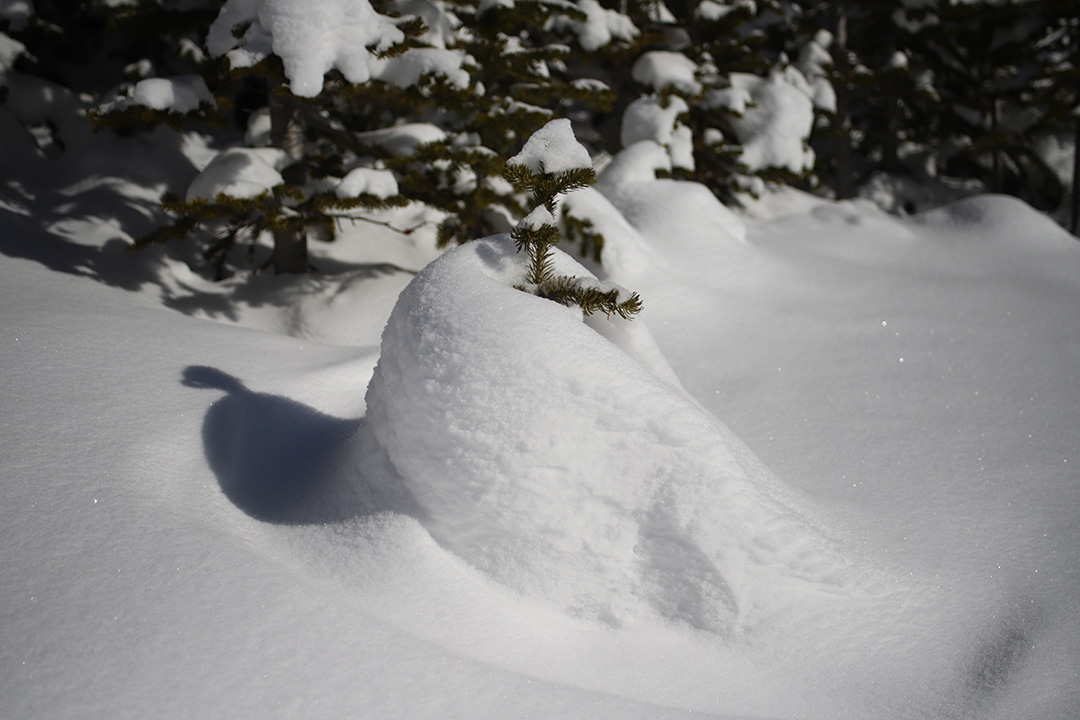
Model scalable to large areas
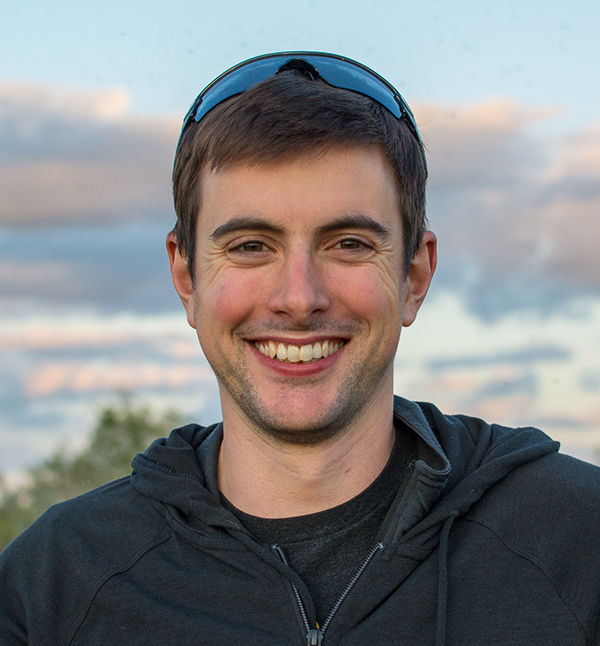
Chris Marsh, a USask post-doctoral fellow who developed the CHM as part of his PhD with the USask Global Institute for Water Security and Department of Geography and Planning, is excited by the potential to scale up the model from a relatively small research domain to larger areas, such as the North American Cordillera (the nearly continuous mountain chain running along the western side of the United States and Canada) or High-mountain Asia (a mountain region that’s home to the world’s biggest collection of glaciers and snow).
“Very large spatial extents are now solvable with a model like this,” said Marsh. “It’s really important for being able to provide estimates for the amount of end-of-winter snow cover in difficult to access mountain areas. Simulations provide a missing piece of the puzzle for helping quantify the amount of water in mountain snow cover.”
Other provinces are already showing interest in using the model for hydrological forecasting, said Pomeroy, and recent discussions with UNESCO (United Nations Educational, Scientific and Cultural Organization) suggest there’s global interest in harnessing its predictive power.
“This will allow for better water management, which is certainly what every water management agency in the world wants right now, with our climate becoming more variable, said Pomeroy. “We’re seeing wild swings in weather and in extreme events, such as droughts and floods. It’s a real challenge for water management, to try to smooth out these extremes, to provide steady supplies for everyone.”
Challenges in building the model
Lead author Vincent Vionnet, now a research scientist with Environment and Climate Change Canada, spent two years with the USask-led Global Water Futures program working on the project. He came up with the design for validating the model, and a strategy for the tricky work of incorporating mountain wind behaviour into the CHM.
As part of their validation, the team was able to toggle on and off the different physical factors in their model—a process called model falsification—to determine how large an influence each had on the accuracy of the information generated.
“We turned off the wind redistribution, we turned off the avalanching,” said Vionnet. “You see a huge decrease in the model’s performance that clearly illustrates the importance of taking into account these processes.”
Costly endeavour
California spends $14 million taking aerial measurements of snowpack in the Sierra Nevada mountains using LiDAR technology, according to Pomeroy.
“They measure it that way because they can’t model it,” said Pomeroy. “We were doing that back in 2007 in the Rockies, but we can’t afford to do it (on a regular basis). We had to figure out how to calculate the snowpack without that information. Sometimes when you don’t have the wealth, you are forced to be smarter.”
This work is supported by a number of funding agencies, including the Canada First Research Excellence Fund (Global Water Futures), and the Natural Sciences and Engineering Research Council of Canada (Discovery Grants).
Article re-posted on .
View original article.