WindMapper
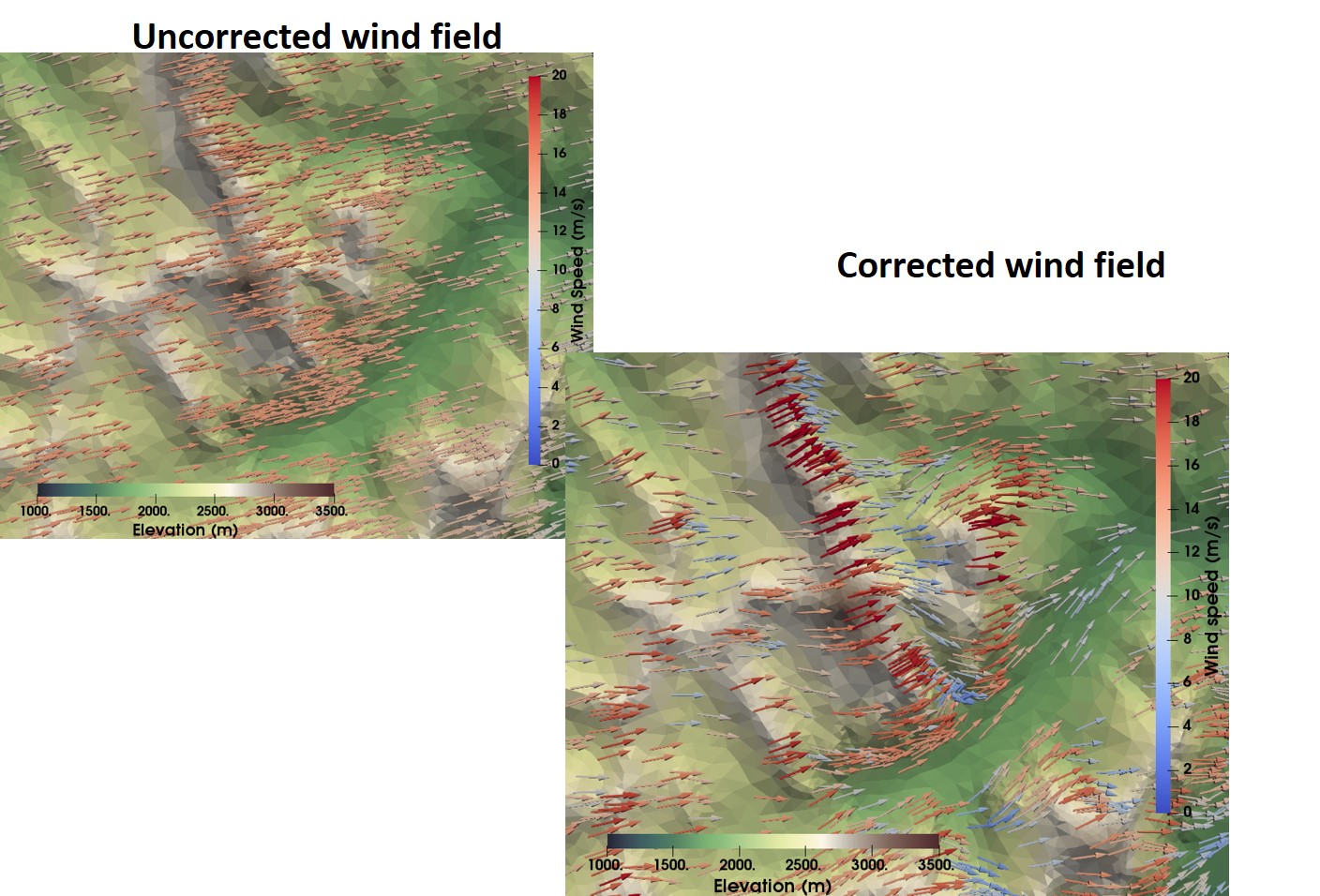
- Wind downscaling method
- Uses pre-computed wind fields from a CFD to downscale winds
- The basis of the wind downscaling in Vionnet, et al. (2020) TCD
- Can be applied to large spatial extents
- https://windmapper.readthedocs.io/en/latest/
Mesher
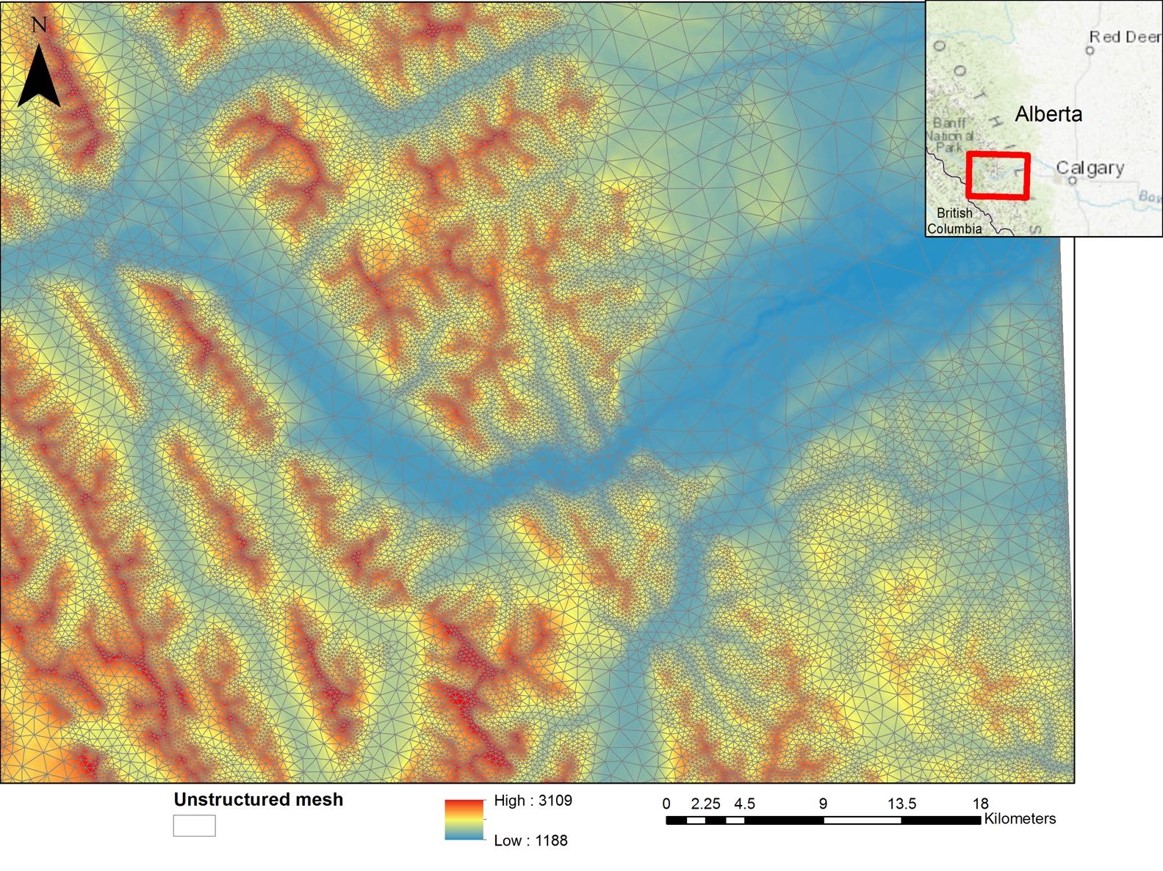
- Mesher generates the unstructured mesh
- Reproducible
- Numerical guarantees on error introduced
- Python/C++, Python configuration
- Test uncertainty from mesh/parameters
- Mesh generation allows assigning raster values to triangles
- Any georeferenced, single-band raster format supported
- Incl VRT files
- Reprojects all input files into a given coordinate system
- Clips all rasters to ensure consistent extent with smallest input raster
- Optionally clips rasters:
- To a netcdf file’s extent
- Good when your domain is larger than input met data
- To some user-specified extent
- Code available on github
EASYMORE
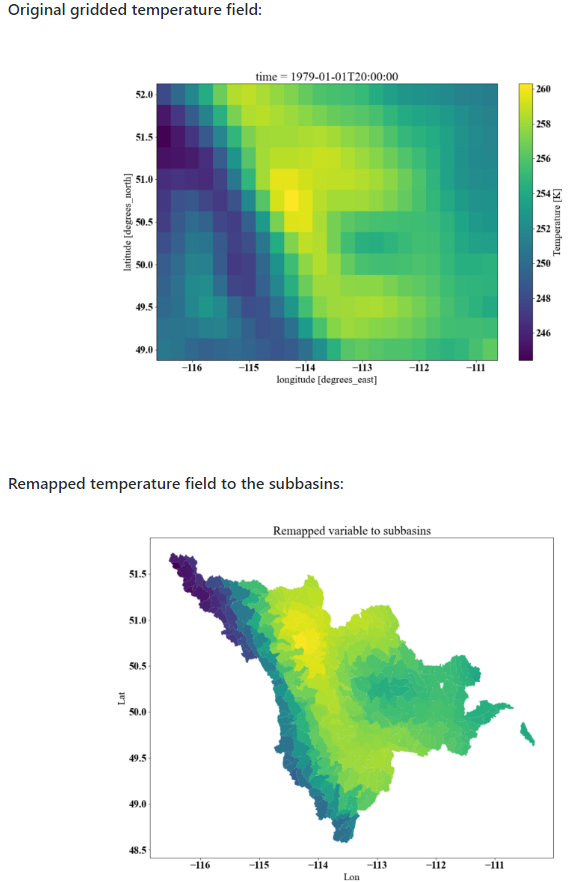
EASYMORE is a collection of functions that allows extraction of the data from a NetCDF file for a given shapefile such as a basin, catchment, points or lines. It can map gridded data or model output to any given shapefile and provide area average for a target variable.
EASYMORE is very efficient as it uses pandas groupby
functionality. Remapping of the entire north American domain from ERA5 with resolution of 0.25 degree to 500,000 subbasins of MERIT-Hydro watershed for 7 variables in 1.2 seconds for one time step (the time varying from device to device and depending on the source netCDF files sizes and their temporal aggregation).
In addition, EASYMORE can perform geospatial processes that are often needed for setting up a hydroligcal or land model. These steps are zonal statistics of raster given a shapefile, zonal statistics of two shapefiles, creation of subbasins and river network topology given hydrologically conditioned DEM such as MERIT-Hydro.
The code can eb used for the following purposes:
- Remapping the relevant forcing variables, such as precipitation or temperature and other variables for the effortless model set up. This transfer can be from Thiessen polygon or gridded data, for example, to computational units, hydrological model for example.
- Remapping the output of a hydrological or land surface model to force another model, such as providing the gridded model output in sub-basin for routing.
- Extraction of single or multiple points from the gridded or irregular data for comparison with gauges data, for example.
- Interpolation to caorser or finer resolutions with full controllability in creating the interpolation rules.
More information is available here: https://github.com/ShervanGharari/EASYMORE
CaSPAr
The Canadian Surface Prediction Archive (CaSPAr) is an archive of numerical weather predictions issued by Environment and Climate Change Canada. Amongst the products archived on a daily basis are five operational numerical weather forecasts, three operational analyses, and one reanalysis product. The products have an hourly to daily temporal and 2.5 km to 50 km spatial resolution. To date, September 2019, the archive contains 394 TB of data while 368 GB of new data are added every night (status: Feb 2020). The data is archived in CF-1.6 compliant NetCDF4 format. The archive is available online since June 2017 and allows users to precisely request data according to their needs, i.e. spatial cropping based on a standard shape or uploaded shapefile of the domain of interest, selection of forecast horizons, variables as well as issue dates. The degree of customization in CaSPAr is a unique feature relative to other publically accessible numerical weather prediction archives and it minimizes user download requirements and local processing time. We benchmark the processing time and required storage of such requests based on 216 test scenarios. We also demonstrate how CaSPAr data can be employed to analyze extreme rainfall events. CaSPAr provides access to data that is fundamental for evaluating numerical weather prediction models and demonstrating the improvement in products such as flood and energy demand forecasting systems.