Thematic Priorities
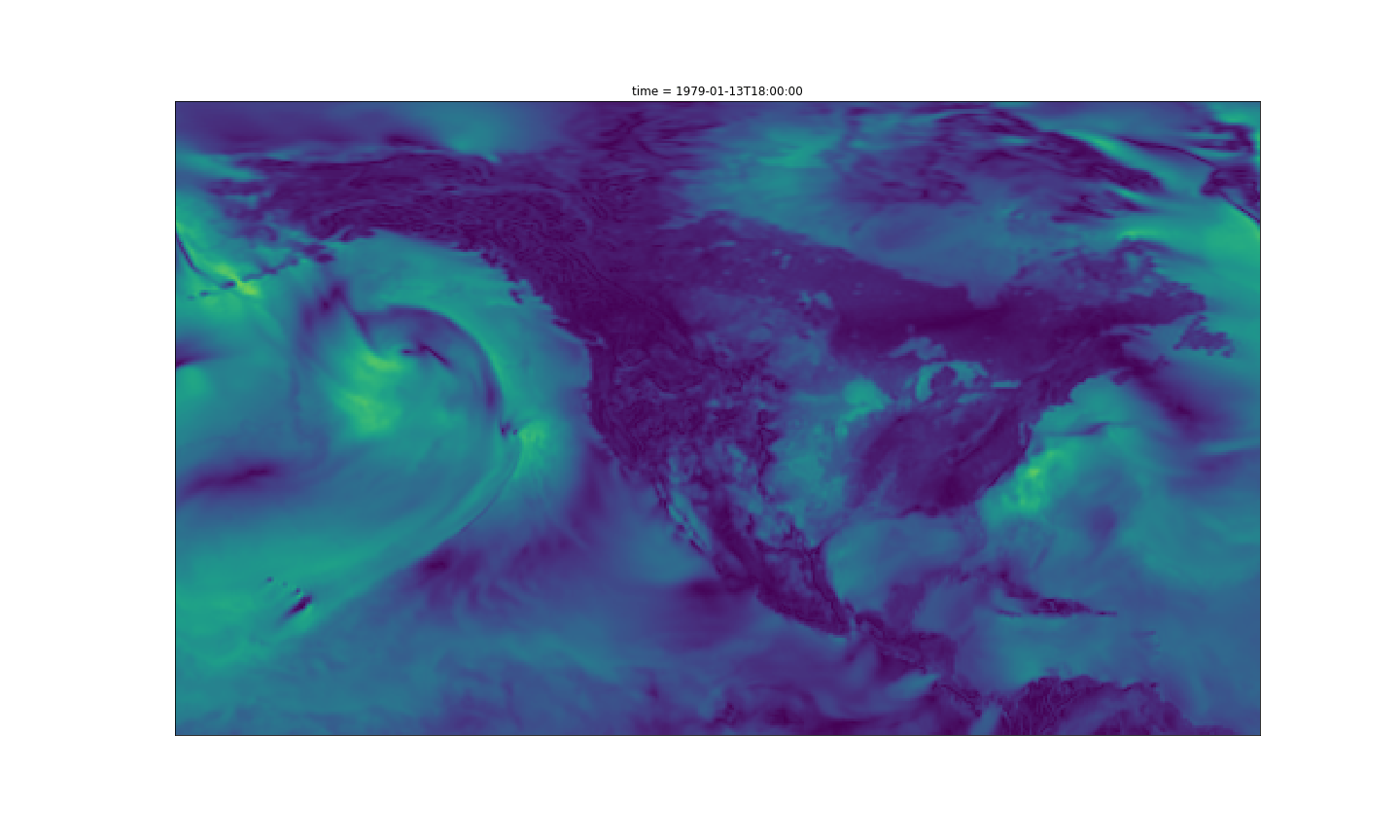
Most spatial meteorological forcing datasets are produced by using station data to adjust Numerical Weather Prediction model reanalyses (primarily WATCH [based on the ECMWF reanalysis] and CaPA [based on GEM]). These datasets are used both to force hydrologic models and to correct biases in large-domain regional climate model simulations (e.g., to correct biases in the pan-Canadian WRF simulations). Work is planned in the following areas:
a. New reanalysis datasets. Environment Canada is currently producing both (i) a new CaPA reanalysis (15-km resolution, 1980-present, should be available in summer 2020); and (ii) a new CaPA ensemble (only run in real time). To build on these datasets, we will develop a new spatial meteorological reanalysis, by (i) combining new regional climate model simulations with existing station networks used in CaPA, along with additional information sources (provincial climate networks, satellite information, etc.), (ii) implement alternative spatial interpolation schemes (e.g., explicitly representing climate gradients), and (iii) quantifying uncertainty. Developing these capabilities will enable rapid development of tailored datasets for critical regions, both in Canada and internationally.
b. New regional climate model simulations. The existing WRF simulations are limited because the boundary forcing provides only weak constraints on the climate within the model domain (partly due to the large domain size). Other options are possible – e.g., alternative configurations of GEM, using small-domain high-resolution instantiations over critical areas (e.g., the Snowcast domain in the upper Bow), periodic re-initialization of GEM using atmospheric reanalyses (e.g., ERA5 or RDRS), various methods to nudge GEM simulations within the model domain.
c. Bias correction. Bias correction of climate model and numerical weather prediction model output.
Faculty Leads
Loading...
Highly Qualified Personnel
Loading...
Collaborators
Loading...